A Mirror or a Fix? Generative AI and the Crisis of Educational Imagination
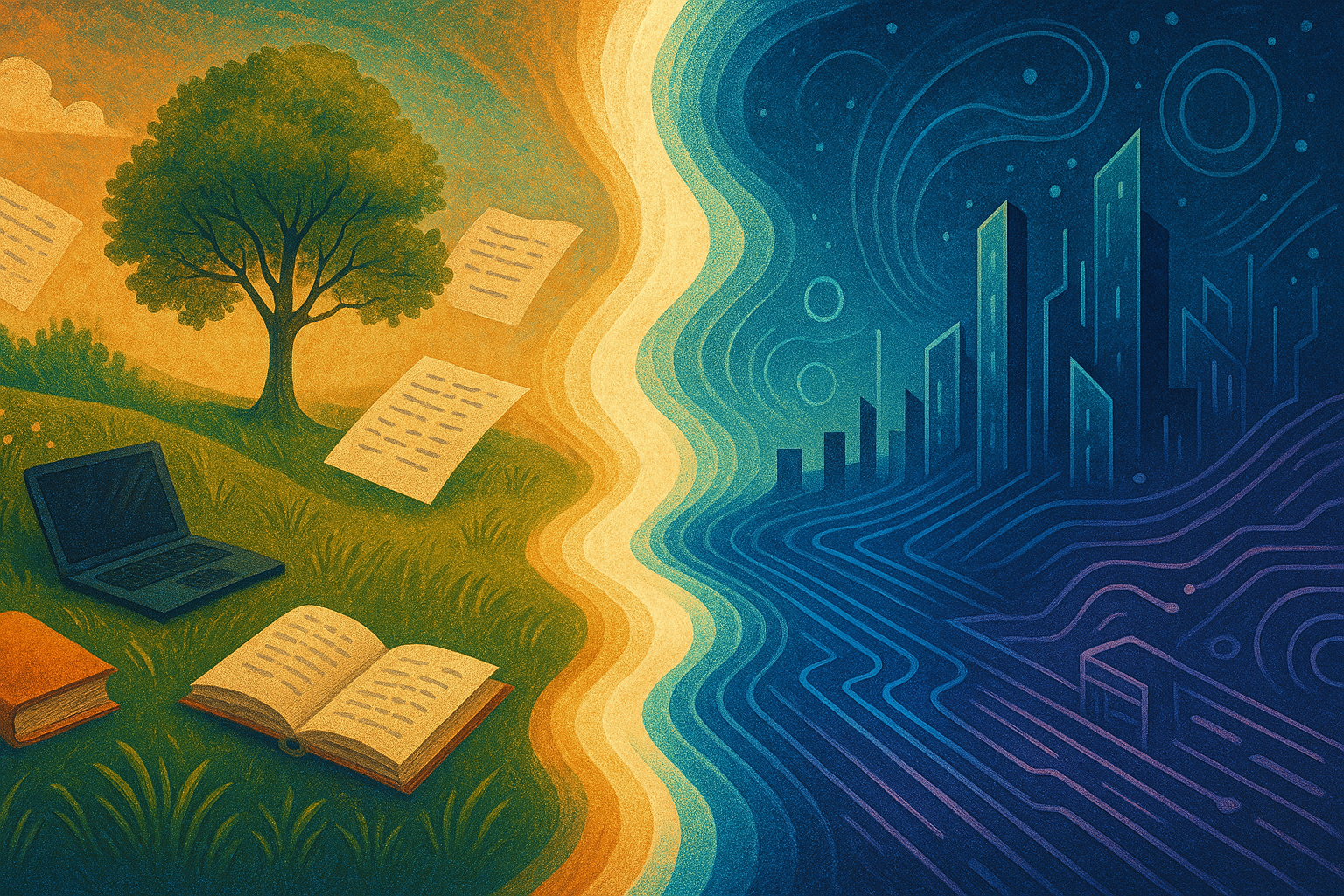
Introduction
As generative AI systems like ChatGPT become more embedded in the life of UK higher education, particularly within Russell Group institutions, the question arises: to what problem is AI the answer? Framed by some as a solution to challenges of workload, assessment, and academic integrity, and by others as a mirror reflecting systemic educational issues, AI is increasingly shaping - not merely responding to - the future of learning.
This post explores the tensions between these two framings: AI as a technological fix, and AI as a pedagogical provocation. Drawing on insights from Helen Beetham and Professor Katie Conrad’s recent podcast conversation, it argues for a turn toward critical AI literacy and reflective, values-driven educational practice.
GenAI as Solution – The Technocratic Response
In many institutions, AI is welcomed as a pragmatic response to growing demands:
- Automating feedback to reduce staff workload.
- Detecting AI-generated plagiarism.
- Enhancing scalability through personalised learning tools.
- Supporting institutional competitiveness via innovation narratives.
These practices align closely with a technocratic, managerial logic. Teaching is seen as a system to be optimised; students as users to be served; learning as a process of efficient content delivery. In this view, AI is a neutral productivity tool - a digital assistant in the service of existing educational structures.
Yet, this framing glosses over deep pedagogical questions. What kinds of knowledge does it privilege? What epistemic assumptions are built into its models? What does it mean when we seek to reduce “friction” in education - when struggle, complexity, and dialogue are the very conditions of learning?
GenAI as Mirror – A Pedagogical Provocation
In contrast, positioning AI as a mirror foregrounds its role in reflecting - and intensifying - underlying tensions in higher education.
AI-generated text challenges the validity of conventional assessments. If a chatbot can produce a passable essay, what does this suggest about the design and purpose of our assignments? As Katie Conrad argues, GenAI systems are “answering questions that educators didn’t ask.” Their outputs expose the assumptions embedded in our teaching: about authorship, originality, expression, and what counts as learning.
This critical framing is grounded in traditions of reflective and critical pedagogy (Freire 1970; hooks 1994), and resists the idea of AI as inevitable or unproblematic. Instead, it invites us to ask:
- Why do we assess the way we do?
- Whose voices are amplified - or erased - by our embrace of AI?
- What educational values are at stake?
Beetham and Conrad suggest that AI can prompt conscientisation - a Freirean awareness of the structures that shape knowledge and power. But only if we are willing to treat AI itself as the object of study - not merely a tool to be adopted, but a text to be read critically.
The Tension: Efficiency vs. Transformation
This leads to a core dialectic:
GenAI as Solution | GenAI as Mirror |
---|---|
Technological fix | Critical reflection |
Operational lens | Pedagogical lens |
Reinforces status quo | Disrupts assumptions |
Prompt engineering | Rights-based questioning |
Russell Group institutions, in particular, face this tension acutely. Their prestige is tied to conventional academic outputs and modes of assessment - yet their legitimacy depends on responding meaningfully to digital disruption. Which framing wins out will shape the trajectory of AI adoption in HE.
Rethinking Assessment and the Right to Voice
Assessment is one of the most urgent sites for reimagining. In the podcast, Conrad critiques the narrowing of academic voice driven by fears of AI misuse. In some settings, students are discouraged from taking creative risks - writing in styles that appear too “non-standard” or emotionally expressive - lest they be flagged for using AI. This flattens diversity and disincentivises experimentation.
Instead, educators might:
- Embrace low-stakes, dialogic assessments.
- Design tasks rooted in personal experience or collaborative inquiry.
- Value ambiguity, iteration, and failure as generative.
Above all, we must reclaim the right to voice - to be heard, to be idiosyncratic, to be human - in an academic system increasingly designed to read, rank, and respond to text as data.
Towards Critical AI Literacy
Conrad and Beetham make a compelling case for critical AI literacy. This goes beyond knowing how to use tools; it entails understanding how and why they work, who they benefit, and what they exclude.
Key elements include:
- Interrogating the training data, assumptions, and ideological frames of AI systems.
- Embedding historical, political, and epistemological perspectives on automation, classification, and labour.
- Designing institutional policies that foreground consultation, transparency, and pedagogical intentionality.
As Beetham warns, many AI “solutions” are being marketed directly to university leaders by vendors whose interests are not aligned with educational values. Educators and students must be equipped to ask: what decisions are being made, by whom, and in whose interests?
Conclusion
To treat GenAI as merely a solution is to ignore the questions it raises about what higher education is - and what it might become. But to engage with it as a mirror is to accept the discomfort of critical reflection and the promise of transformation.
The future of education will not be determined by how efficiently we use AI, but by whether we can resist its instrumentalisation long enough to imagine other possibilities.
Reflective Prompts
- What would it mean to make GenAI the object of study in your course, rather than a tool for delivery?
- Are we using AI to solve problems without questioning the design that produced them?
- What forms of assessment would be worth doing, even if AI could replicate them?
- How can we centre student voice, vulnerability, and difference in the age of GenAI?
- What rights - of authorship, transparency, consent - must we defend as educators and learners?
References
Benjamin, R. (2019). Race after technology abolitionist tools for the new Jim code / Ruha Benjamin. Medford, MA: Polity.
Beetham, H. and Conrad, K. (2025). Imperfect Offerings: Talking about AI in Education [Podcast]. Available at https://helenbeetham.substack.com
Biggs, J.B. (John B. et al. (2022). Teaching for quality learning at university. Fifth edition / John Biggs, Catherine Tang, Gregor Kennedy. Maidenhead: Open University Press.
Conrad, K. (2023). Blueprint for an AI Bill of Rights in Education. [Blog] Critical AI. Available at https://criticalai.org
Freire, P. (2018). Pedagogy of the oppressed / Paulo Freire ; translated by Myra Bergman Ramos ; with an introduction by Donaldo Macedo and an afterword by Ira Shor. 50th anniversary edition. New York: Bloomsbury Academic.
hooks, bell. (1994). Teaching to Transgress Education As the Practice of Freedom. 1st ed. Oxford: Taylor & Francis Group.
Knox, J. (2020) ’Artificial intelligence and education in China’, Learning, Media and Technology, 45(3), pp. 298–311. doi https://doi.org/10.1080/17439884.2020.1754236
Selwyn, N 2019, Should robots replace teachers? AI and the Future of Education. 1st edn, Polity Press, Cambridge UK.
Williamson, B., Eynon, R. and Potter, J. (2020). Pandemic politics, pedagogies and practices: digital technologies and distance education during the coronavirus emergency. Learning, media and technology, 45(2), pp.107–114.